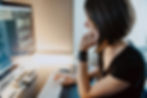
In 2018, only 20% of the students earning Bachelor's Degrees in Computer Science were women (Partovi & Hendrickson, 2020). However, the number of women in Computer Science has fluctuated up and down through the years. In the 1960s, computer jobs were devalued so women worked the majority of them. Then, in the 1970s, computer jobs became more prestigious resulting in men taking over the roles (Brewer, 2017). Not only that, but women were also slowly forced out of the computer industry as personal computers became more widely available in the 1980s because they were advertised to men (Henn, 2014). Therefore, despite women having an early dominance in the computer industry, ultimately men took over, resulting in the gender gap we see today in computer science careers.
The gender gap in computer science exists both within industry and academia. According to the New York Times, the world’s reliance on computer algorithms and artificial intelligence has “led to concerns that the tech industry’s dominantly white and male work forces are building biases into the technology underlying those systems” (Metz, 2019). It’s essential that the employees of tech companies are diverse. This helps avoid bias. In the U.S. in 2020, 68% of Google’s employees were men. Additionally, 51.7% of their employees are white and 41.9% of their employees Asian, which is a common minority seen in the tech fields (Google Diversity Report, 2020). That means only 10.4% of Google’s employees are Black, Latinx, or Native American. In 2019, these minorities were found to make up 31.2% of the overall U.S. population (Frey, 2020). Google is one of the most powerful companies in the world, so its lack of diversity has everlasting impacts.
Knowing that certain populations in the U.S. are underrepresented in technology fields is a problem because of the unforeseen bias that results in the technology that is created. The majority of the time, this is an unconscious bias that results from certain groups of people not being represented or thought about in the making of technology. A bias has been found in studies on the accuracy of different facial recognition softwares. A NIST study in 2019 found that there were higher rates of false positives for African American women than other groups (Henderson, 2020). Not only that, but they found in general there were more false positives in people of African or Asian descent and more for women than men (Grother et al., 2019). The implications of these studies are staggering. Facial recognition software is used to make arrests, so if some groups are more likely to have a false positive, that could lead to false arrests. It is essential that we ensure there is diversity in technology companies to ensure the technology we create is unbiased and fair for everyone.
In Invisible Women, Caroline Criado Perez investigates a phenomenon called the gender data gap (Criado Perez, 2019). Many technologies are created with men in mind, which is most likely a result of the technologies being created predominately by men. One such example is the newer versions of the iPhone, which are “too large for the average women’s hand” (Wimmer, 2020). Additionally, Machine Learning and Artificial Intelligence are trained on initial datasets, so if the datasets that a model is trained on is missing information about certain groups, the model won’t work as well on those groups. This is seen with facial recognition not working as well on certain ethnic groups. As technology continues to get more advanced, it is essential that we ensure there is equal representation in who is writing the code, as well as in other STEM fields.
Sources
Partovi, H., & Hendrickson, K. (2020, May 11). Women computer science graduates finally surpass record set 17 years ago, but percentages lag behind. Retrieved December 29, 2020, from https://codeorg.medium.com/women-computer-science-graduates-finally-surpass-record-set-17-years-ago-20a79a76275
Brewer, K. (2017, August 10). How the tech industry wrote women out of history. Retrieved January 01, 2021, from https://www.theguardian.com/careers/2017/aug/10/how-the-tech-industry-wrote-women-out-of-history
Henn, S. (2014, October 21). When Women Stopped Coding. Retrieved January 01, 2021, from https://www.npr.org/sections/money/2014/10/21/357629765/when-women-stopped-coding
Metz, C. (2019, June 21). The Gender Gap in Computer Science Research Won't Close for 100 Years. Retrieved January 01, 2021, from https://www.nytimes.com/2019/06/21/technology/gender-gap-tech-computer-science.html
Google Diversity Report. (2020). Retrieved January 01, 2021, from https://diversity.google/annual-report/
Frey, W. H. (2020, July 16). The nation is diversifying even faster than predicted, according to new census data. Retrieved January 01, 2021, from https://www.brookings.edu/research/new-census-data-shows-the-nation-is-diversifying-even-faster-than-predicted/
Henderson, S. (2020, May 18). NIST Study Evaluates Effects of Race, Age, Sex on Face Recognition Software. Retrieved January 01, 2021, from https://www.nist.gov/news-events/news/2019/12/nist-study-evaluates-effects-race-age-sex-face-recognition-software
Grother, P., Ngan, M., & Hanaoka, K. (2019). Face recognition vendor test part 3:. doi:10.6028/nist.ir.8280
Criado-Perez, C. (2019). Invisible women: Exposing data bias in a world designed for men. London: Vintage.